The growing risks of unstructured data in the AI era
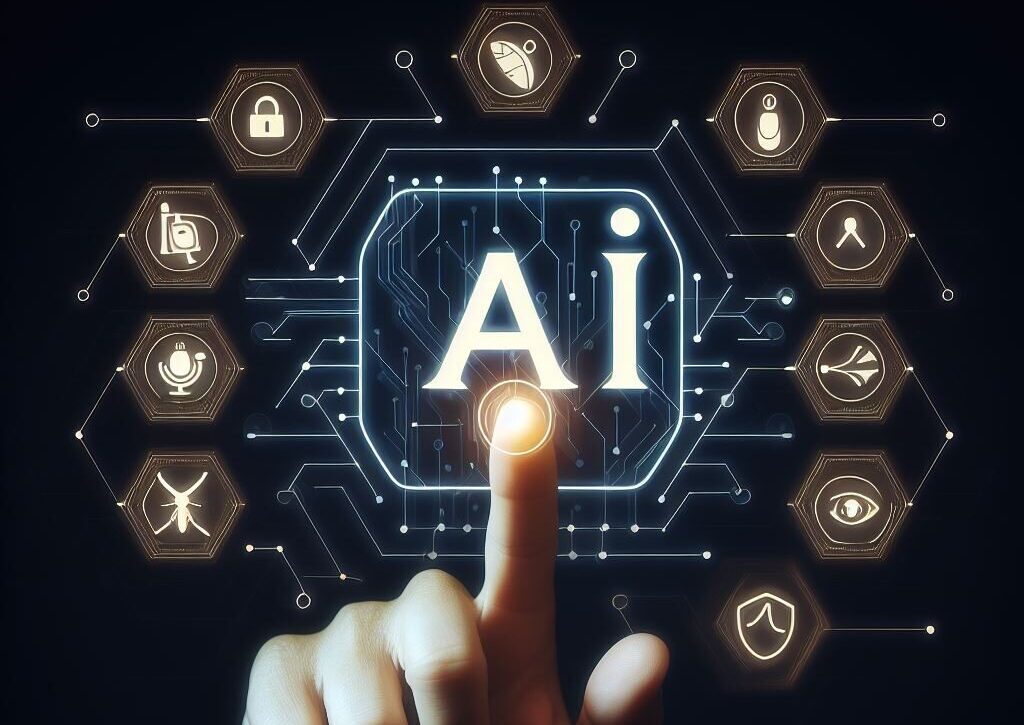
As of 2024, two years after the world was first introduced to ChatGPT—an implementation of generative AI and one of the most popular forms of AI, it still remains one of the fastest-growing technological advancements in the world. From Healthcare to Finance to transportation and Education, AI has quickly become an essential part of our everyday lives.
At the core of this AI revolution is Data. Data is the fuel that powers AI models and systems. However, all data is not the same.
A significant portion of the data driving AI today is unstructured data. Files, chats, texts, emails, audio files, images, and videos all fall under unstructured data. While this unstructured data could become immensely useful, it also comes with some risks that are becoming increasingly obvious in today’s AI era.
Unpacking Unstructured Data
To fully understand the risks in unstructured data, we must first understand what it is.
Unstructured data refers to information that does not fit into a predefined format or organizational model. They tend to come in different forms and are more difficult to analyze. Examples of unstructured data include customer reviews on Yelp, rows of text on iMessage, or even that 25-minute video on YouTube. Files stored across different organizational systems, such as emails in Microsoft Outlook or enterprise platforms, project documents in shared drives, or media files in cloud storage, are also common examples of unstructured data. Simply put, if it’s written or created by humans, it is likely a type of unstructured data.
Right now, unstructured data is estimated to make up about 80% of all the data generated worldwide, and it is more so within organizations!
For organizations, unstructured data often constitutes an even more significant portion, as emails, documents, media files, and other non-tabular data dominate enterprise storage systems, creating both opportunities and challenges for data management and utilization
Furthermore, according to the International Data Corporation(IDC), by 2025, the overwhelming majority of the projected 163 zettabytes(163 trillion gigabytes) of the global datasphere will be unstructured data!
Fun fact: This article contains unstructured data!
That said, AI systems thrive on unstructured data. Due to its share size, a lot of valuable insights can be gotten from it. The more popular large language models (LLMs), like Google’s Gemini 1.5 and OpenAI’s GPT- 4o, were both trained on unstructured data. But it’s not all roses and sunshine!
The very traits that make unstructured data an invaluable goldmine for AI advancements also make it quite risky.
The Risks of Using Unstructured Data in Generative AI Processes
As unstructured data continues to grow, so do the risks associated with its management and use. But how bad can it get? Here are 5 key risks of unstructured data and real-world consequences of said risks.
1. Privacy Concerns
Due to its sheer size and relatively messy nature, unstructured data often contain sensitive information— like private messages, images, emails, and audio files. And while many organizations try their best to clean out this private information, it can still be challenging to separate private information from unstructured data with 100% accuracy, leading to increased privacy concerns for users.
That said, privacy concerns don’t just affect users; they also affect organizations as a whole. Organizations that fail to implement the proper security systems for unstructured data risk violating privacy regulations like the European General Data Protection Regulation (GDPR). And although it might be unintentional, companies that violate these regulations end up with negative business consequences like huge fines and questionable reputations.
A real-world example of this occurred in 2022 when Facebook(now Meta) had to pay a fine of about $277 Million for a privacy breach that exposed the personal data of millions of users. Again, in 2023, Ireland's Data Protection Commission fined Meta Platforms approximately $1.3 billion for violating the General Data Protection Regulation (GDPR) by exposing user information to potential unauthorized access.
2. AI Bias
AI is often referred to as objective, inclusive or fair. However, when AI is trained on unstructured data, it can sometimes lead to amplified biases. These biases in AI might sometimes be so minute, they are difficult to spot, and sometimes so obvious that the entire system needs an overhaul.
Biased languages in historical texts or underrepresentation in images can reinforce stereotypes in AI outputs. This could particularly prove risky in the hiring sector ,where recruiters are becoming more dependent on AI in their selection process.
Another real-world example of this occurred in 2018 when world-renowned Amazon Inc. had to scrap its AI recruiting engine because it was discovered that the AI preferred hiring men over women. Turns out the unstructured data on which the AI was trained had a historical bias against women.
3. Quality Issues
Unlike structured data that fits neatly into rows and columns, Unstructured data is often noisy, incomplete, and inconsistent. Unstructured data can also be ambiguous and sometimes hypothetical, making it prone to errors during analysis or AI model training.
Mislabeled images, poorly transcribed audio, or wrongly spelled words could all negatively impact an AI’s ability to make accurate decisions.
A glaring example of the risks in unstructured data could be seen when IBM’s Watson Health AI received a lot of negative criticism. While it was initially praised as the next big thing in healthcare, its reliance on unstructured data led to a host of problems.
Professionals began observing that the AI was recommending inaccurate, and sometimes dangerous treatments to cancer patients. It was later discovered that the risky assessments were a result of the AI being trained on hypothetical data— instead of real client data.
4. Security Vulnerabilities
Due to its vast, varied, and decentralized nature, unstructured data can sometimes be a prime target for cyberattacks. While structured data is easier to encrypt and secure using a lot of standard safety and security protocols, unstructured data is much harder to monitor and protect.
Hackers tend to exploit vulnerabilities in unstructured data systems, stealing sensitive information or corrupting data to deceive AI models. Attacks on AI systems—like subtly modifying an image to mislead a computer vision model, could pose a huge security threat.
A prime example of this occurred in 2020 when a Tesla computer vision system was tricked by a small alteration in road signs. Researchers discovered that by adding inconspicuous stickers to a stop sign, they could trick Tesla's autopilot system into misinterpreting it as a speed limit sign, causing the vehicle to accelerate instead of halting and consequently leading to terrible road crashes.
Unstructured data risks also extend beyond autonomous vehicles. In 2021, a cybersecurity firm showed how minor audio manipulations could trick voice-activated AI assistants like Amazon Alexa and Google Assistant into executing unauthorized commands.
These altered audio inputs were undetectable to human ears and exploited unstructured data vulnerabilities in natural language processing (NLP) systems. The potential risks ranged from minor privacy invasions to serious unauthorized financial transactions
5. Model Poisoning
The final risk we’ll be looking at is Model poisoning. Model poisoning is a significant threat to AI systems. Hackers intentionally introduce false or misleading information into unstructured data when training the AI model. They do this to manipulate the outcomes of the model.
One method involves placing misinformation through channels like emails, which, if incorporated into the training data, can degrade the model's performance or cause it to generate incorrect outputs.
While specific documented cases of data poisoning through email misinformation are limited, the broader concept has been observed in various AI applications. For instance, attackers have manipulated training data in AI models to introduce biases or vulnerabilities, leading to compromised decision-making processes.
The Silver Lining
In conclusion, despite the risks and challenges, unstructured data holds incredible potential when handled correctly. The risks associated with it should serve as a call to action for industry leaders in the AI space to create smarter solutions to manage data, build better AI systems, and improve security.
The good news is that one such solution exists with Acumen AI. Using AcumenAI, you can easily avoid exposure to the risks of unstructured data in AI.
Reach out to us, and let’s mitigate your risks.
About Author
Let's get your data AI-ready! Hit us up
Get a free report to see how ChatGPT and other Large Language Models (LLMs) are being utilized within your network.